This brief fieldtrip report will be a short one, since I was in the Danish capital in all just two-and-a-half days, and was busy with work mostly: four public speaking appearances, a slew of meetings, a dinner with clients… oh – and there was also my 56th birthday, which I managed to celebrate a little. As for micro-tourism, we’d two or three hours set aside for this in the itinerary, but, as luck would have it, it was raining cats and dogs and a gale was blowing at the allotted time: result – no micro-tourism; result of that – little to write here I’m afraid folks…
So let’s see. Ah yes – I’ll start with a few… curious photos of me up on stage during my first presentation:
My next speech was to a Danish CISO club. It was in a very swish, clearly very old, classic-imperial hall with Roman columns and wood-paneled walls adorned with grand old paintings depicting significant (probably local/national) historical events. Very impressive. Thing is… I don’t know the name of the place! So if there’s anyone among you, dear readers, who could let me know (by checking the pic below) – in the comments, I’d be much obliged. I’d love to read up on the place’s history…
Here’s the pic of the hall, taken from the back:
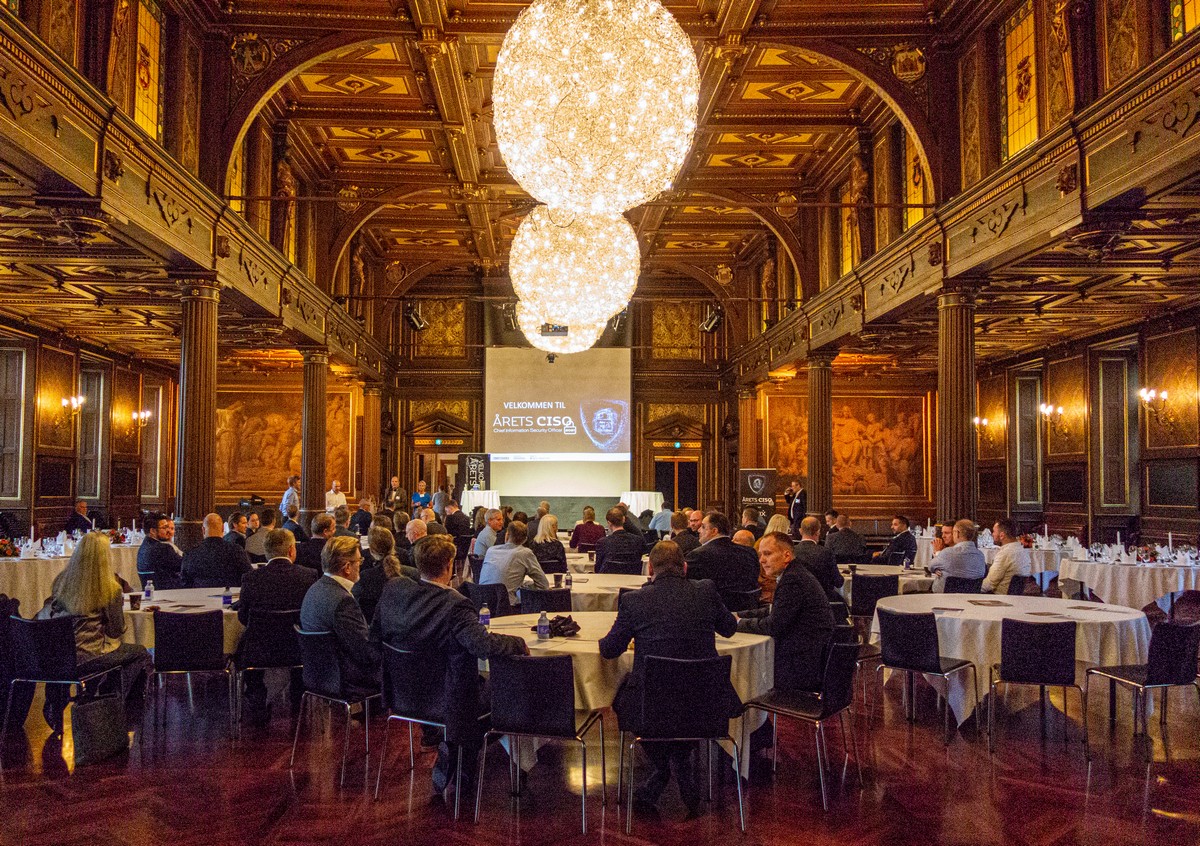
When I took that, in 15 minutes I’d be up on stage addressing the audience. So how did it go? Splendidly! I said what needed saying (geopolitics get outta here + some of our success stories), and I joshed a bit (as I do; I can’t help it:), and the crowd… they weren’t just smiling, weren’t just chuckling… they were roaring with whoops of laughter! So much for skeptical! So, like I say – splendid ).
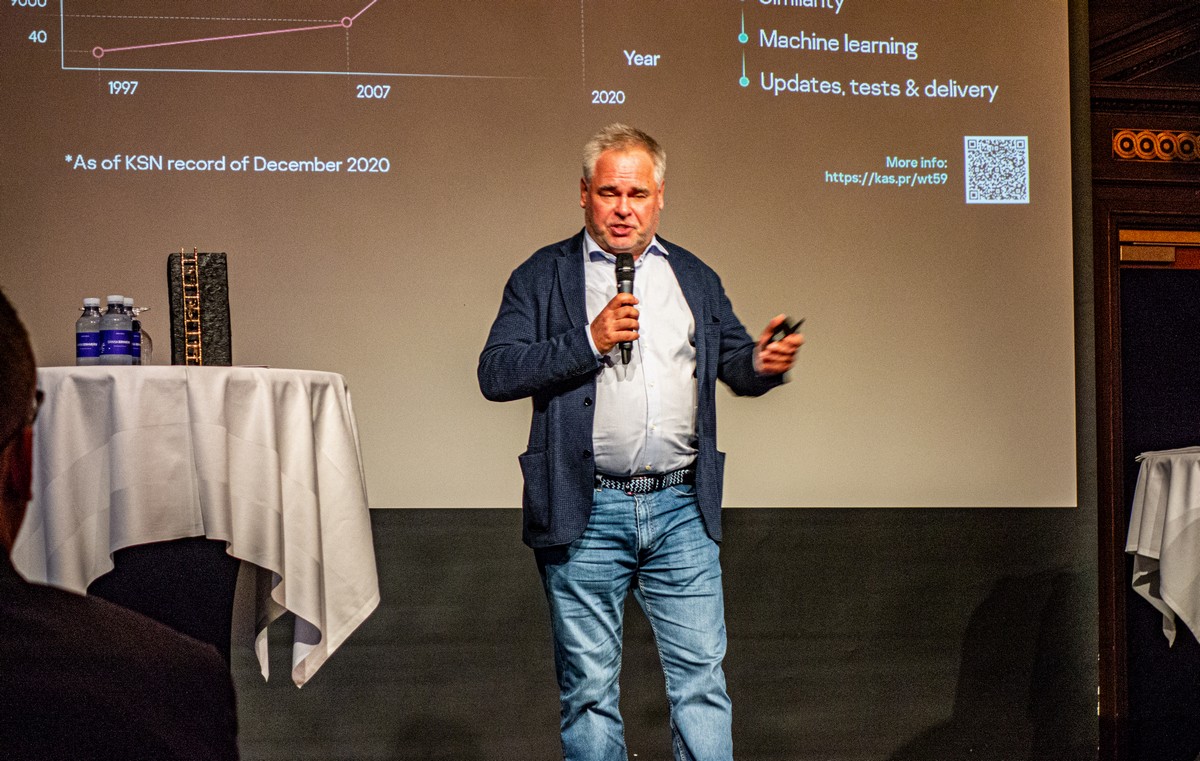
Sorry for the poor quality photos. My fault – I took the wrong camera 😕.
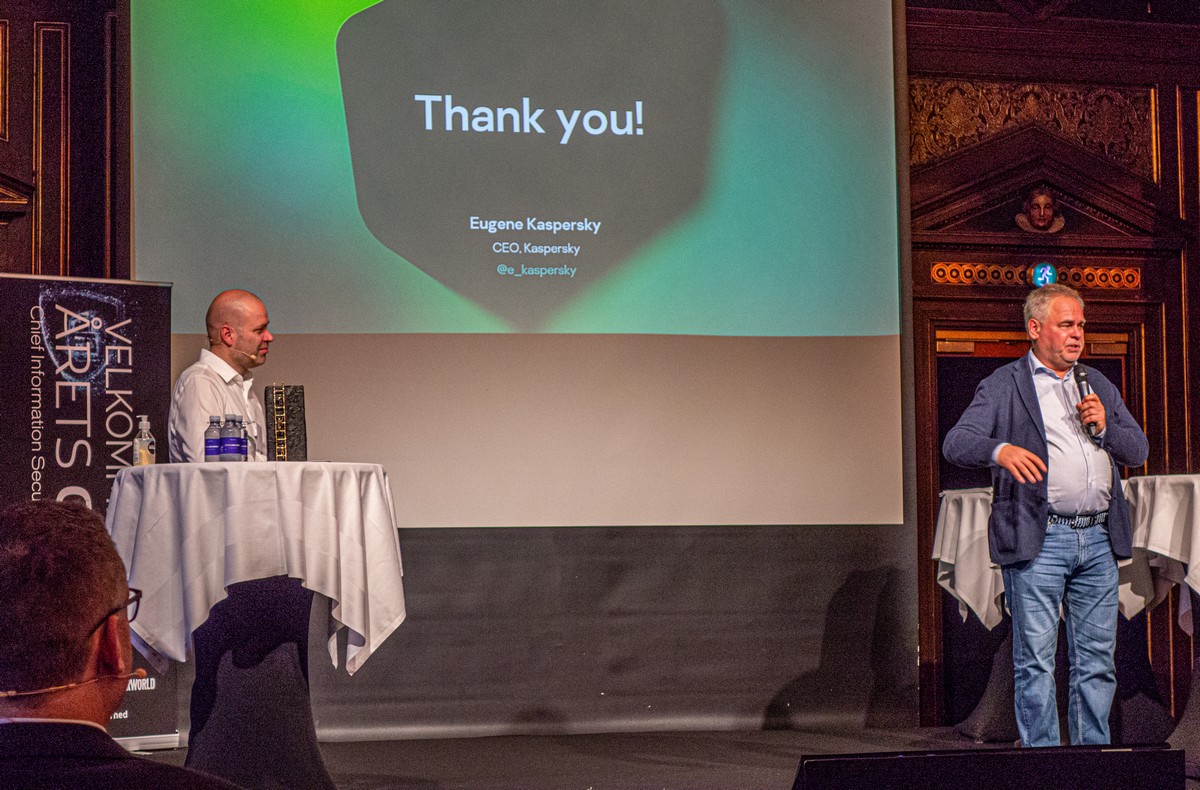
After my speech, I noticed a wooden… contraption, which, I was told, was once used for ‘collecting dues for the army’. No complaints there: Russia and Denmark have never been at war with each other ).
Here’s me and a good journalist from a good magazine. Check out our expressions and body language!
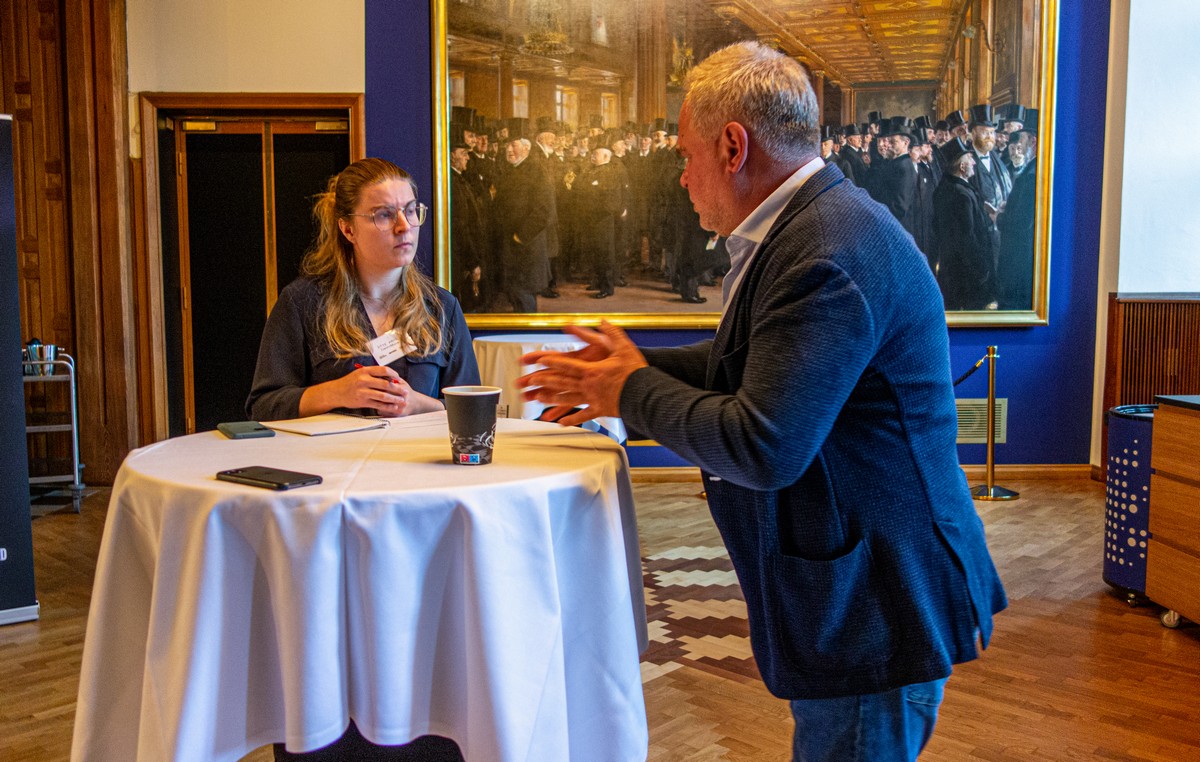
Btw – you see the painting in the background? All those top-hatted gentlemen – in the very hall I’d just given a speech in… Didn’t politicians all dress like that 200 years ago? Hmmm – I think I’m getting warmer as to the identity of this place: a former parliament building? Maybe even the government’s former premises (surely not current)?… Ok, I’m warmer; but could someone please… bring me up to the boil?…
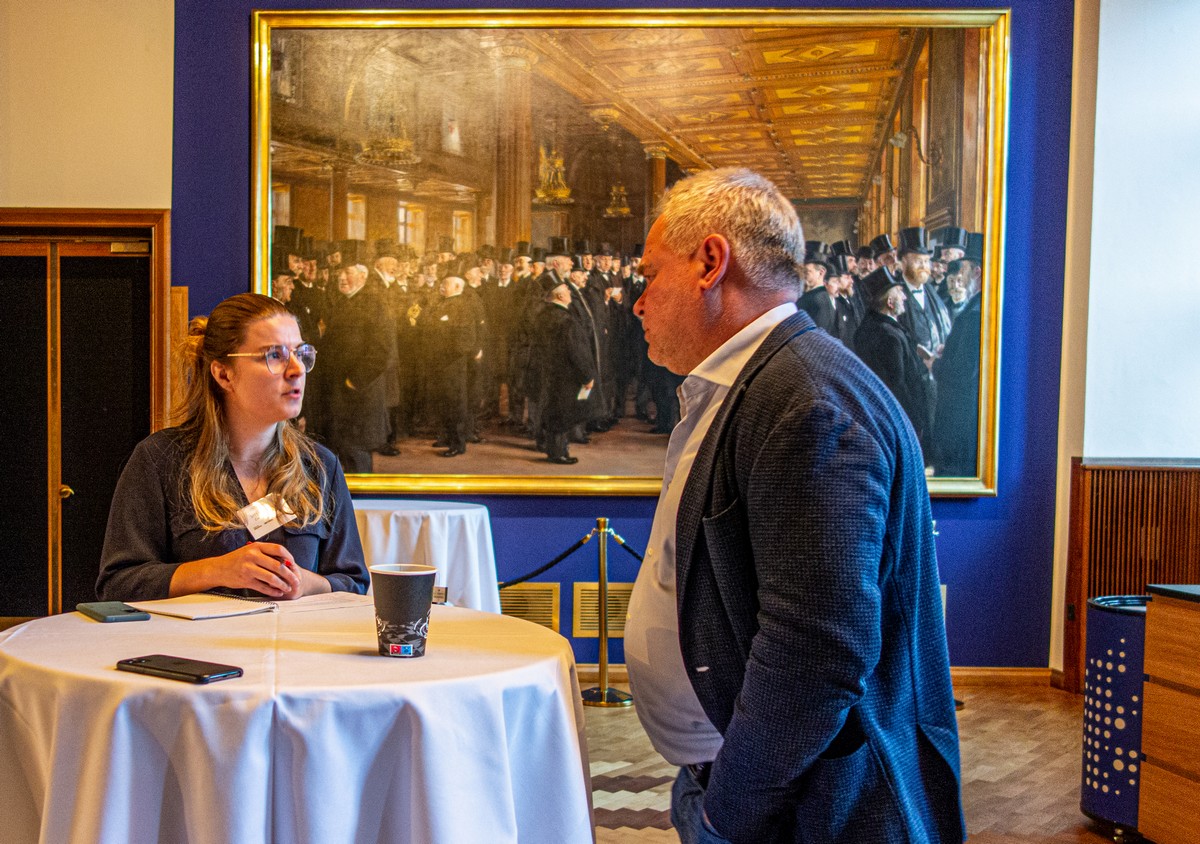